The rapid growth of e-commerce has unfortunately been accompanied by a surge in online fraud. Traditional security measures, like simple rule-based systems, struggle to keep pace with the increasingly sophisticated tactics employed by fraudsters. This is where machine learning (ML) steps in as a critical tool, offering a dynamic and adaptive approach to protecting online payments. Unlike static methods, ML algorithms can learn from vast amounts of transaction data, identifying patterns and anomalies that would otherwise be undetectable. This capability is essential in a landscape where fraud techniques evolve rapidly.
Machine Learning: A Sentinel Against Fraud
Machine learning excels at fraud detection through sophisticated pattern recognition. These algorithms analyze various data points associated with each transaction, such as purchase history, location, device information, and spending habits. By establishing a baseline of normal behavior for each customer, the system can quickly flag transactions that deviate from that established profile. For instance, a sudden purchase from an unusual location or a large order that is inconsistent with past behavior could raise a red flag and trigger further investigation. This proactive approach drastically reduces the likelihood of successful fraudulent transactions. Choosing the payment e-commerce solutions is essential here.
Preventing Costly Chargebacks
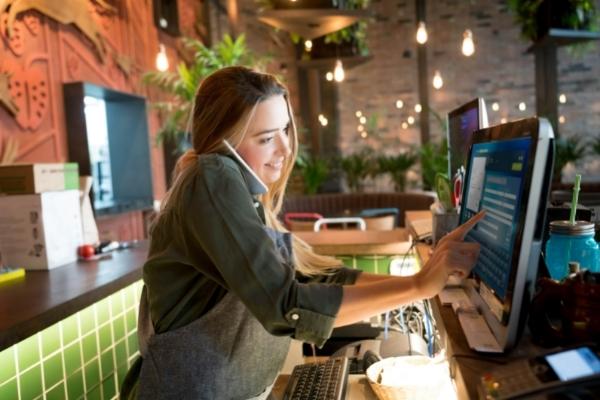
Chargebacks, where customers dispute a transaction and demand a refund, represent a significant financial burden for e-commerce businesses. Often, chargebacks happen due to genuine fraud, or even when a customer might claim they didn’t authorize a purchase, whether it was from accidental or intentional use of their information. Machine learning, again, helps to mitigate this problem. By accurately identifying fraudulent transactions before they are completed, ML-based systems drastically reduce the number of illegitimate charges requiring chargeback processing. Also, by providing detailed analysis to companies, machine learning gives them more data and background to help make their chargeback investigations easier and more accurate.
Safeguarding Customer Data Through Anonymization
Beyond fraud detection, machine learning is instrumental in protecting sensitive customer data during online transactions. Techniques such as data anonymization, where sensitive elements like credit card numbers and personal addresses are replaced with random identifiers, are increasingly employed alongside ML algorithms. This allows the algorithms to process valuable data for analysis and model training without exposing personally identifiable information. Doing so enhances privacy and builds trust with customers by demonstrating a commitment to data security. Additionally, advanced encryption techniques are often incorporated into machine learning processes to ensure that sensitive data remains secure even during transit or processing.
The Future of E-Commerce Security
Machine learning is not a static solution, but rather a constantly evolving tool in the battle against online fraud. As algorithms continue to improve and new technologies emerge, the ability to detect and prevent fraudulent transactions will only become more refined. E-commerce platforms that invest in robust ML-based security systems are not only safeguarding themselves from financial losses but are also building greater customer trust and confidence in the safety of online shopping, and securing the future of online payments.